Esmorís, A.M., Weiser, H., Winiwarter, L., Cabaleiro, J.C. & Höfle, B. (2024): Deep learning with simulated laser scanning data for 3D point cloud classification. ISPRS Journal of Photogrammetry and Remote Sensing. Vol. 215, pp. 192-213. DOI: 10.1016/j.isprsjprs.2024.06.018
3D point clouds acquired by laser scanning are invaluable for the analysis of geographic phenomena. To extract information from the raw point clouds, semantic labelling is often required. The labelling task can be solved in an automatic way using deep learning methods, but this requires large amounts of training data which are costly to acquire and annotate.
In this work, we investigate the use of simulated virtual laser scanning (VLS) data for training deep learning models, which are used to classify real data. We validate the method for two use cases: Multi-class urban classification and binary leaf-wood classification.
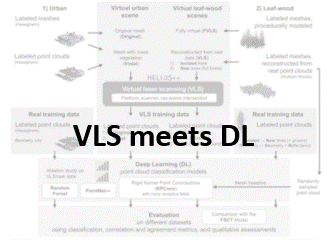
In an ablation study, we compare the performance of VLS and real training datasets on many models with different amounts of annotated points.
For leaf-wood classification, our deep learning model trained purely on VLS data produces results comparable to models trained on real data when evaluated on real data, with just 1 % less overall accuracy (93.7 % with VLS training data, 94.7 % with real training data). For the urban classification with multiple, unbalanced classes, the gap between real and simulated training data seems to be larger and more work is needed in terms of scene modeling to improve informative value of VLS data.
Point clouds generated by LiDAR simulation perform clearly better than point clouds generated by simple random sampling of points on the surface of our input meshes. This is reflected both in superior classification results and in a better match of the histograms of geometric features.
General strengths of the VLS-DL approach are:
- Large amounts of diverse laser scanning training data can be generated quickly and at low cost.
- Simulation configurations can be adapted so that the virtual training data match the targeted real data.
- The whole workflow can be automated, e.g., through procedural scene modelling, and can even be implemented as a single Python workflow for VLS simulation and DL.
Related work
For the virtual laser scanning, we use HELIOS++, the Heidelberg LiDAR Operations Simulator, a flexible and powerful open-source laser scanning simulation software which is hosted on GitHub: 3dgeo-heidelberg/helios
Winiwarter, L., Esmorís, A.M., Weiser, H., Anders, K., Martínez Sanchez, J., Searle, M., Höfle, B. (2022): Virtual laser scanning with HELIOS++: A novel take on ray tracing-based simulation of topographic full-waveform 3D laser scanning. Remote Sensing of Environment, 269, https://doi.org/10.1016/j.rse.2021.112772
Funding
This research was funded by the Deutsche Forschungsgemeinschaft (DFG), German Research Foundation, by the projects SYSSIFOSS (Grant Number: 411263134) and VirtuaLearn3D (Grant Number: 496418931).
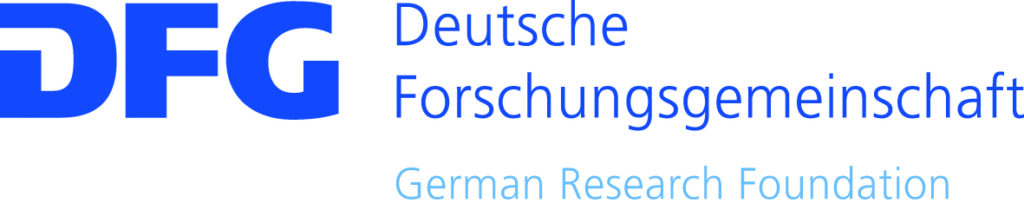
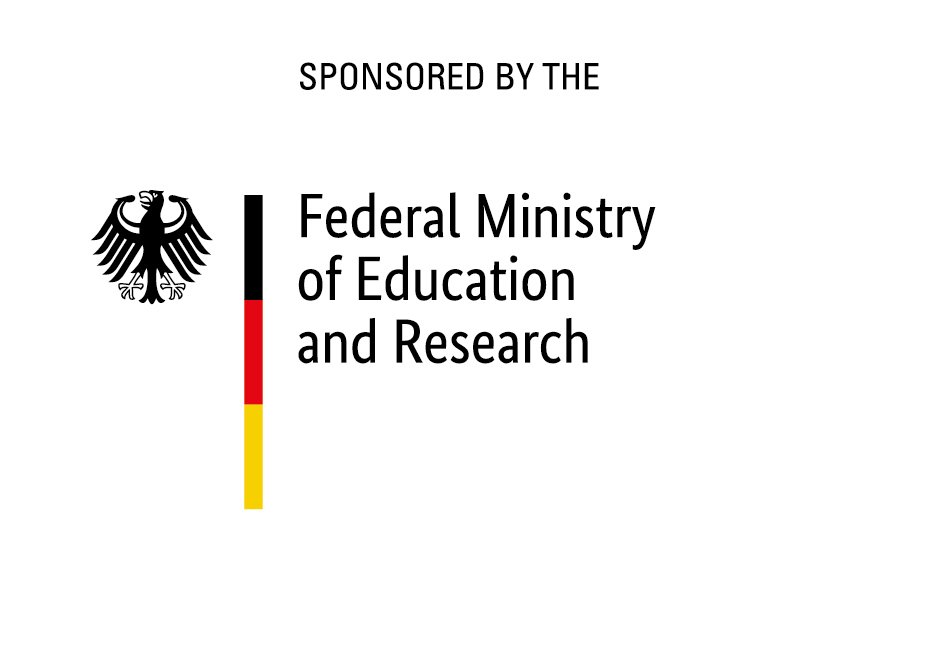
This work has also received financial support from the Consellería de Cultura, Educación e Ordenación Universitaria (accreditation ED431C 2022/16 and accreditation ED431G-2019/04) and the European Regional Development Fund (ERDF), which acknowledges the CiTIUS-Research Center in Intelligent Technologies of the University of Santiago de Compostela as a Research Center of the Galician University System, and the Ministry of Economy and Competitiveness, Government of Spain (Grant Number PID2019-104834GB-I00 and PID2022-141623NB-I00).
The many deep learning experiments computed on the FinisTerrae-III supercomputer were possible thanks to the CESGA (Galician supercomputing center). Diverse experiments were also possible thanks to the data curators of the Hessigheim and Wytham Woods datasets and the manually labelled leaf-wood datasets.
The authors gratefully acknowledge support by the state of Baden-Württemberg through bwHPC and the German Research Foundation (DFG) through grant INST 35/1597-1 FUGG. Furthermore, the authors acknowledge the data storage service SDS@hd supported by the Ministry of Science, Research and the Arts Baden-Württemberg (MWK) and the German Research Foundation (DFG) through grant INST 35/1503-1 FUGG.
Open Access funding enabled and organized by Projekt DEAL.