In great collaboration with colleagues from Karlsruhe (DE), Vienna (AT), Brno (CZ), Leipzig (DE), Raszyn (PL), and Berlin (DE), we published a paper investigating approaches to improve LiDAR-based biomass models when only limited sample plots with field data are available. The main work was carried out by PhD student Jannika Schäfer (IFGG, Karlsruhe Institute of Technology), using the forest growth simulator Forest Factory, the laser scanning simulator HELIOS++, a couple of awesome datasets (incl. pytreedb) and a lot of brainpower.
Background
Airborne laser scanning (ALS) data are increasingly used to predict forest biomass over large areas. Biomass information cannot be derived directly from airborne laser scanning data; therefore, field measurements of forest plots are required to build regression models that use ALS metrics as predictors. However, field measurements are time consuming and costly, especially when field plots are remote or difficult to access. There is thus an economic interest to keep the number of field plots small.
Method
We tested whether simulated laser scanning data from virtual forest plots could be used to train biomass models, thereby reducing the amount of field measurements required. We compared the performance of models that were trained with
- simulated data only
- a combination of simulated and real data
- real data collected from different study sites
- real data collected from the same study site the model was applied to
We also investigated whether using a best-matching subset of the simulated data, rather than using all the simulated data, improved model performance.
Models were tested on four forest sites located in Poland, the Czech Republic, and Canada. Model performance was assessed by root mean square error (RMSE), coefficient of determination (r2), and mean error (ME) of observed and predicted biomass.
Results
We found that models trained solely on simulated data did not achieve the accuracy of models trained on real data (RMSE increase of 52–122 %, r2 decrease of 4–18 %). However, model performance improved when only a subset of the simulated data was used (RMSE increase of 21–118 %, r2 decrease of 5–14 % compared to the real data model), albeit differences in model performance when using the best-matching subset compared to using a randomly selected subset were small. Using simulated data for model training always resulted in a strong underprediction of biomass. Extending sparse real training datasets with simulated data decreased RMSE and increased r2, as long as no more than 12–346 real training samples were available, depending on the study site. For three of the four study sites, models trained with real data collected from other sites outperformed models trained with simulated data and RMSE and r2 were similar to models trained with data from the respective sites.
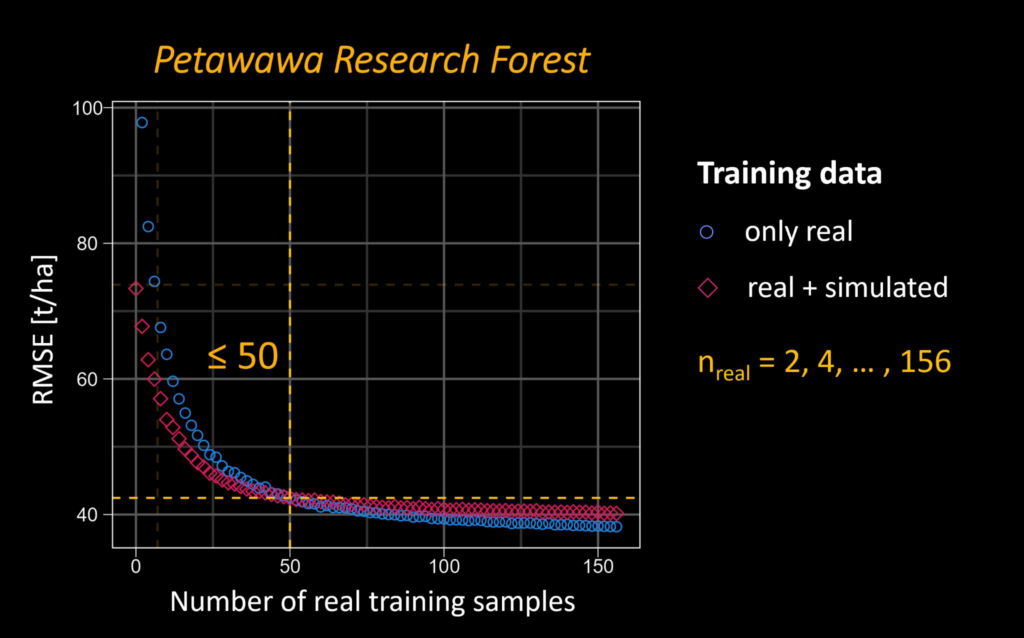
When less than 51 real training samples are available, adding simulated data leads to a better biomass model for the Petawawa Research Forest in terms of RMSE
(Figure: Jannika Schäfer).
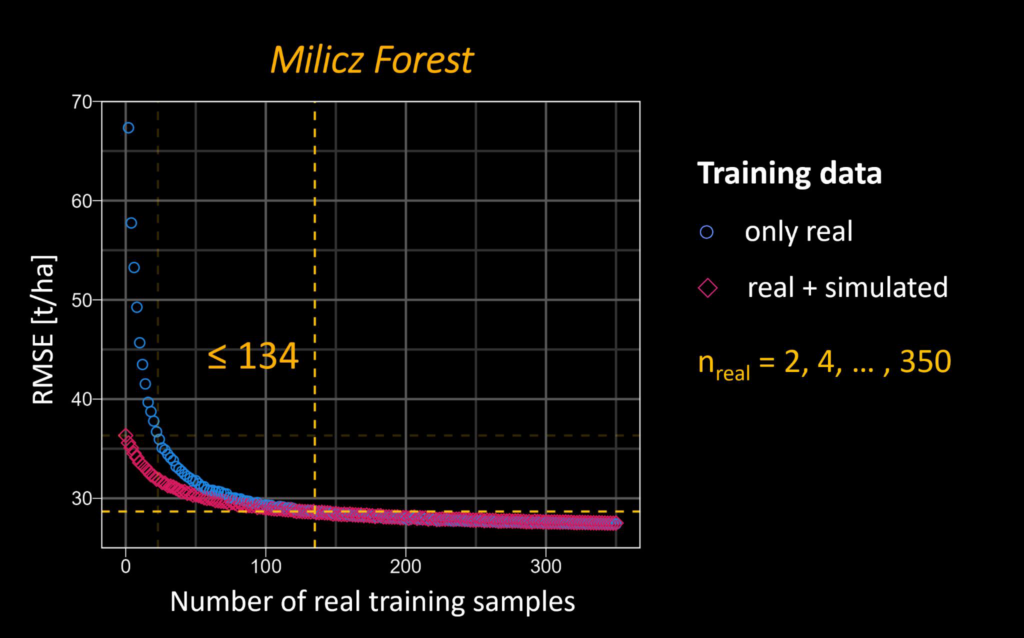
For the Milicz forest, the threshold is even higher. Only when more than 134 real training samples are used, the simulated data cease to benefit
(Figure: Jannika Schäfer).
Conclusion
Our results suggest that simulated data cannot yet replace real data, but may be useful in some locations to extend training datasets when there is a limited amount of real data available.
Reference
Schäfer, J., Winiwarter, L., Weiser, H., Novotný, J., Höfle, B., Schmidtlein, S., Henniger, H., Krok, G., Stereńczak, K. & Fassnacht, F. E. (2023): Assessing the potential of synthetic and ex situ airborne laser scanning and ground plot data to train forest biomass models. Forestry: An International Journal of Forest Research, cpad061, pp. 1-19. https://doi.org/10.1093/forestry/cpad061
Funding
This work was supported by the Deutsche Forschungsgemeinschaft (DFG, German Research Foundation) in the frame of the project SYSSIFOSS – 411263134 / 2019 2022; by the Polish State Forests National Forest Holding in the frame of the project “Development of the method of forest inventory using the results of the REMBIOFOR project” (Project No. 500463, agreement No. EO.271.3.12.2019); and by the National Centre for Research and Development (Poland) in the frame of the REMBIOFOR project “Remote sensing-based assessment of woody biomass and carbon storage in forests” as part of the BIOSTRATEG programme (Agreement No. BIOSTRATEG1/267755/4/NCBR/2015).
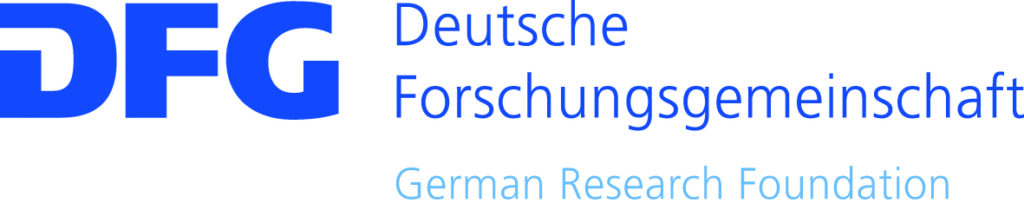