OpenStreetMap (OSM) has been intensively used to support humanitarian aid activities, especially in the Global South. Its data availability in the Global South has been greatly improved via recent humanitarian mapping campaigns and due to the efforts of local communities. However, large rural areas are still incompletely mapped. The timely provision of map data is often essential for the work of humanitarian actors in the case of disaster preparation or disaster response. In this context, volunteers or humanitarian organizations often ask where do we need to map in OpenStreetMap next?
To answer such questions, we recently developed the Missing OSM Maps service to identify OSM missing built-up areas within countries that recently suffered from natural disasters (e.g., flood, tsunami, volcano eruption, etc.). The objective is to establish an end-to-end framework for fast and accurate unmapped human settlements localization and detection by integrating heterogeneous geospatial datasets, such as volunteered geographical information (VGI), social media (geotagged tweets), and remote sensing (RS)

satellite images.
Currently, the processed study areas range from Mozambique (hit by Cyclone Idai in 2019), and India (hit by Cyclone Fani in 2019), to the Kingdom of Tonga (affected by Hunga Tonga eruption in 2022), See Figure 1. The Missing OSM Maps provides an intuitive way of exploring OSM missing built-up area, which consists of mainly 4 steps (Figure 2):
- Step1: What is already in OpenStreetMap? – First, users can check how many features, specifically buildings, have been mapped by OSM volunteers by setting Satellite Opacity and DeepVGI Opacity both to zero.
- Step2: What can we see from remote sensing satellite images? – Next, users can further check whether there are real human settlements over the study areas. In order to look into the satellite images, you might set Satellite Opacity to one, and leave DeepVGI Opacity as zero.
- Step3: What can we get from DeepVGI built-up estimation from RS? – In this step, we demonstrate the DeepVGI automatic building detection results, which are generated based on our deep learning models together with very high-resolution satellite images. For more detail, you might want to check out our related publication.
- Step4: Can we even count the number of human settlements from RS? – In addition to the binary estimation of built-up areas, the perdition of the total number of human settlements within each satellite image tile is also available. (still beta version)
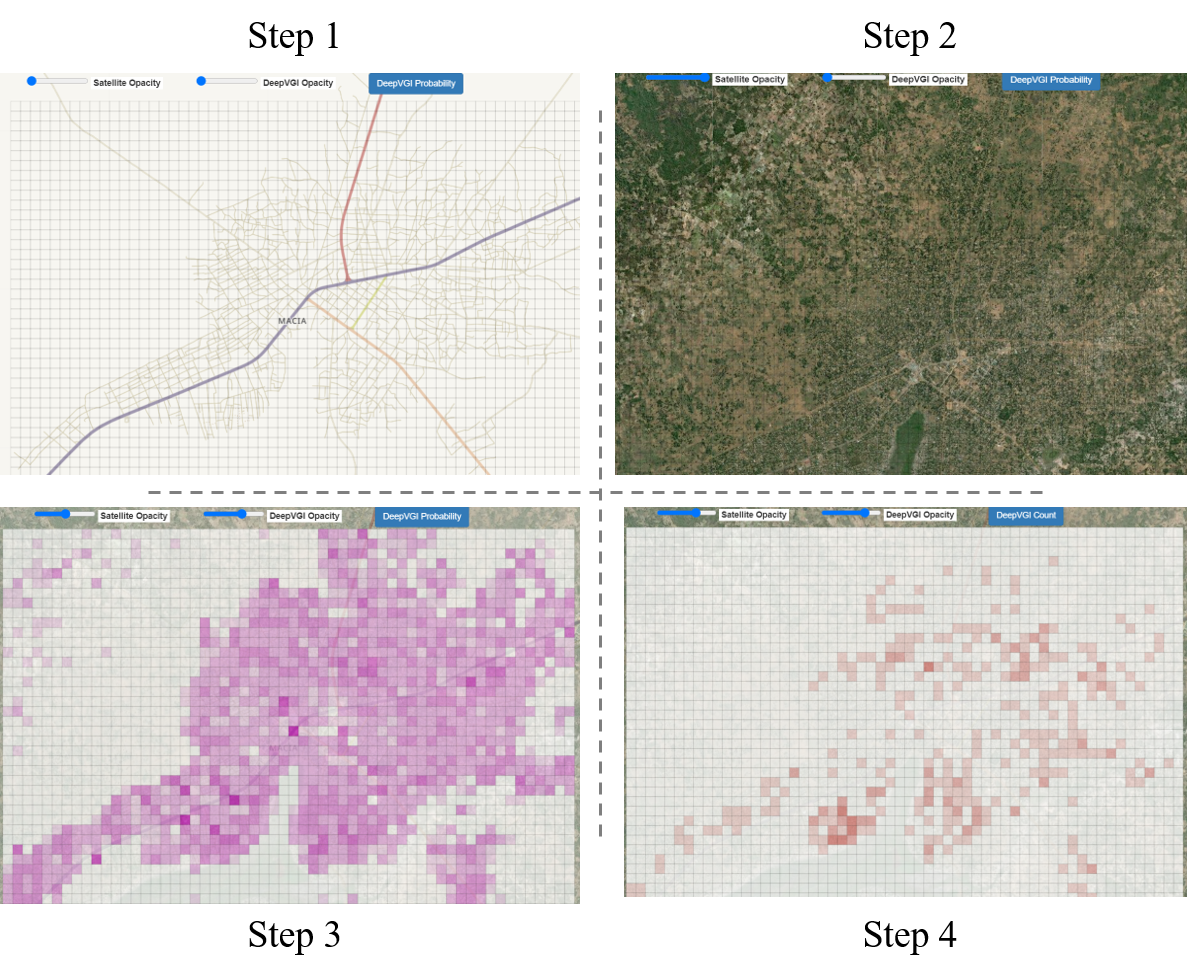
In our DeepVGI project, we are interested in combining deep learning technology with crowdsourcing VGI data for real-world problems. The concept and method used to create the Missing OSM Maps were proposed in Herfort et al. 2019 and Li et al. 2019. Moreover, the walk through of “Automatic building detection with ohsome2label and Tensorflow” is openly available on our GIScience GitHub.
In future work, we want to further develop this method and extend this service, with which we aim to support better and faster humanitarian mapping from both a machine-assisted mapping perspective and an OSM data quality assessment perspective. Stay tuned for our future updates and have fun with the Missing OSM Maps!
The Missing OSM Maps service is available here: https://missingosm.geog.uni-heidelberg.de/
Methods used:
- Herfort, B., Li, H., Fendrich, S., Lautenbach, S., Zipf, A. (2019): Mapping Human Settlements with Higher Accuracy and Less Volunteer Efforts by Combining Crowdsourcing and Deep Learning. Remote Sensing 11(15), 1799. https://doi.org/10.3390/rs11151799
- Li, H., Herfort, B., Huang, W., Zia, M., and Zipf, A. (2020): Exploration of OpenStreetMap Missing Built-up Areas using Twitter Hierarchical Clustering and Deep Learning in Mozambique. ISPRS Journal of Photogrammetry and Remote Sensing. https://doi.org/10.1016/j.isprsjprs.2020.05.007
Previous related work:
- Herfort, B., Lautenbach, S., Porto de Albuquerque, J., Anderson, J., Zipf, A. (2021): The evolution of humanitarian mapping within the OpenStreetMap community. Scientific Reports 11, 3037 (2021).
DOI: 10.1038/s41598-021-82404-z - Pisl, J., Li, H., Lautenbach, S., Herfort, B., and Zipf, A. (2021): Detecting OpenStreetMap missing buildings by transferring pre-trained deep neural networks, AGILE GIScience Ser., 2, 39, https://doi.org/10.5194/agile-giss-2-39-2021.
- Li, H., Herfort, B., Zipf, A. (2019): Estimating OpenStreetMap Missing Built-up Areas using Pre-trained Deep Neural Networks. Proceedings of the 22nd AGILE Conference on Geographic Information Science, Limassol, Cyprus.
- Zipf, A, Chen, J. (2017): Humanitarian Mapping with Deep Learning and Volunteered Geographic Information. BIG 2017 (BigData Innovators Gathering). Perth. co-located with WWW2017.
- Chen, J., Y. Zhou, A. Zipf and H. Fan (2018): Deep Learning from Multiple Crowds: A Case Study of Humanitarian Mapping. IEEE Transactions on Geoscience and Remote Sensing (TGRS). 1-10. https://doi.org/10.1109/TGRS.2018.2868748
- Scholz, S., Knight, P., Eckle, M., Marx, S., Zipf, A. (2018): Volunteered Geographic Information for Disaster Risk Reduction: The Missing Maps Approach and Its Potential within the Red Cross and Red Crescent Movement. Remote Sens., 10(8), 1239, doi: 10.3390/rs10081239.
- Barron, C., Neis, P. & Zipf, A. (2013): A Comprehensive Framework for Intrinsic OpenStreetMap Quality Analysis. Transactions in GIS, DOI: 10.1111/tgis.12073.