In our efforts to improve change detection and quantification in time series of 3D point clouds, we have published yet another milestone. Our paper, titled M3C2-EP: Pushing the limits of 3D topographic point cloud change detection by error propagation, presents a novel method to better separate noise from actual change in point clouds sensed with laser scanners. To achieve this, we combine knowledge on the accuracy of the sensors with knowledge on the uncertainty introduced by aligning two datasets before change detection.
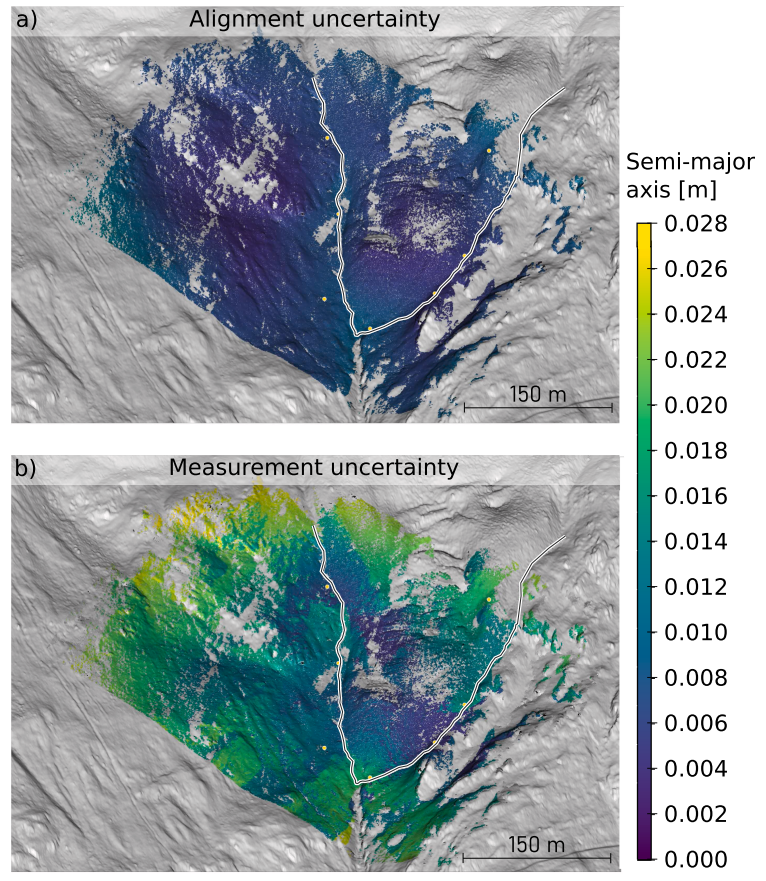
In contrast to the current state-of-the-art, this allows us a more sensible and adaptive threshold to identify areas where change has been recorded.
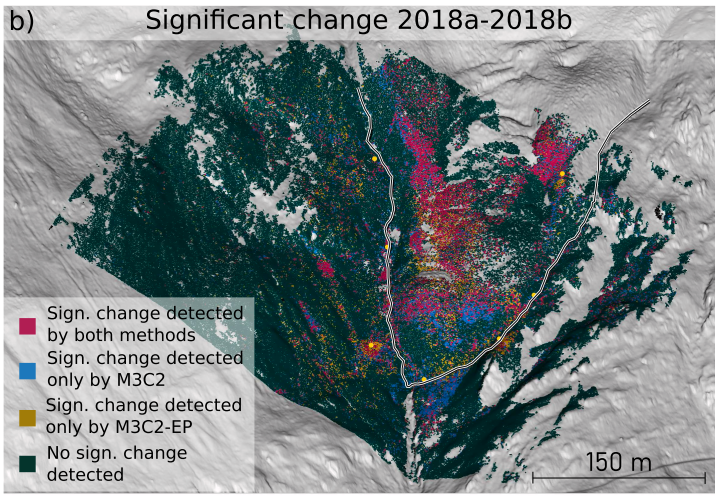
The paper is available under open access and CC-BY 4.0 clause here: https://doi.org/10.1016/j.isprsjprs.2021.06.011, the accompanying data and code are available on heiData: https://doi.org/10.11588/data/XHYB10.
Winiwarter, L., Anders, K., & Höfle, B. (2021). M3C2-EP: Pushing the limits of 3D topographic point cloud change detection by error propagation. ISPRS Journal of Photogrammetry and Remote Sensing, 178, 240–258. https://doi.org/10.1016/j.isprsjprs.2021.06.011
M3C2-EP adds another important method to the toolbox of 4D point cloud processing methods developed by the 3DGeo group.